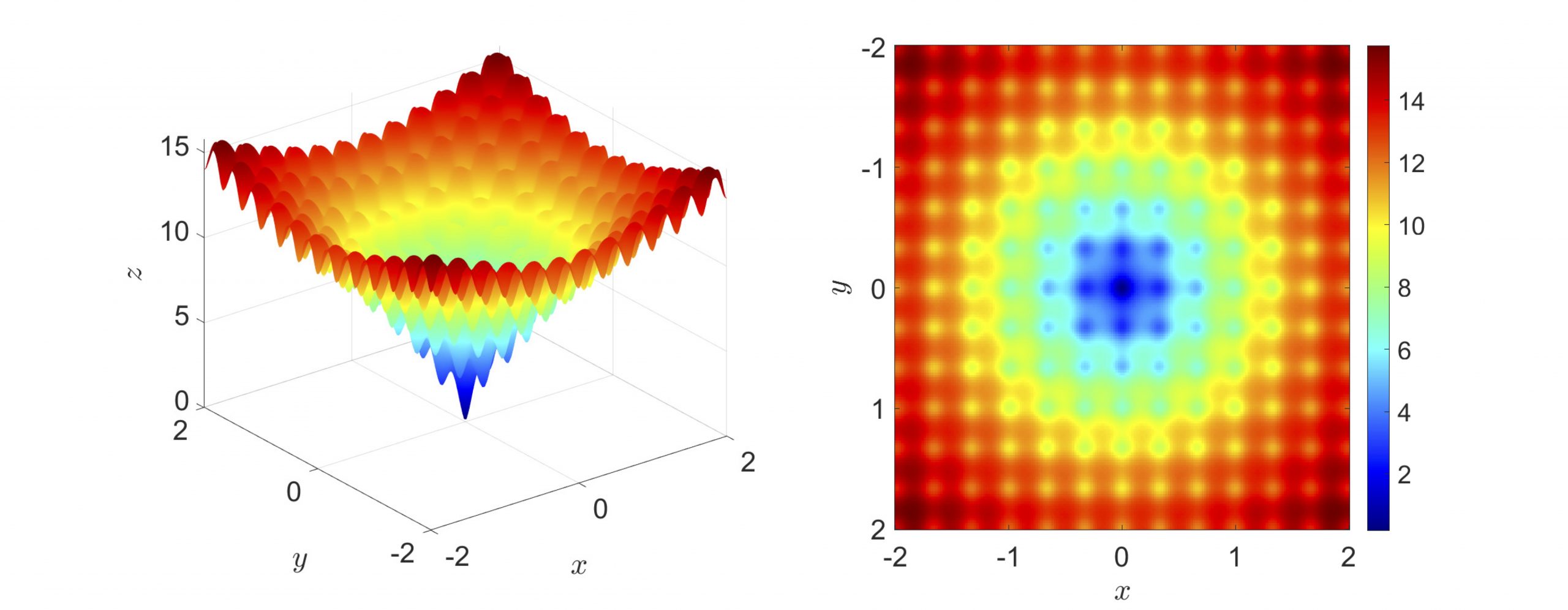
The papers “Minimax Control of Ambiguous Linear Stochastic Systems Using the Wasserstein Metric“, and “A Stochastic Consensus Method for Nonconvex Optimization on the Stiefel Manifold” have been accepted to the IEEE Conference on Decision and Control (CDC). The first paper proposes a novel linear-quadratic control method that effectively deals with “uncertainty about uncertainty”. We derived a Riccati equation and characterized the condition for closed-loop stability. More interestingly, we identified a theoretical connection to the classical H_\infty robust control in the sense that our method is a distributional generalization of the H_\infty approach. The second paper introduces an interesting consensus system of stochastic differential equations that can be used to find global optima of nonconvex optimization problems over the Stiefel manifold.
Minimax Control of Ambiguous Linear Stochastic Systems Using the Wasserstein Metric
by Kihyun Kim, and Insoon Yang
Abstract: In this paper, we propose a minimax linear- quadratic control method to address the issue of inaccurate distribution information in practical stochastic systems. To construct a control policy that is robust against errors in an empirical distribution of uncertainty, our method is to adopt an adversary, which selects the worst-case distribution. To systematically adjust the conservativeness of our method, the opponent receives a penalty proportional to the amount, measured with the Wasserstein metric, of deviation from the empirical distribution. In the finite-horizon case, using a Riccati equation, we derive a closed-form expression of the unique optimal policy and the opponent’s policy that generates the worst-case distribution. This result is then extended to the infinite-horizon setting by identifying conditions under which the Riccati recursion converges to the unique positive semi-definite solution to an associated algebraic Riccati equation (ARE). The resulting optimal policy is shown to stabilize the expected value of the system state under the worst-case distribution. We also discuss that our method can be interpreted as a distributional generalization of the H∞-method.
A Stochastic Consensus Method for Nonconvex Optimization on the Stiefel Manifold
by Jeongho Kim, Myeongju Kang, Dohyun Kim, Seung-Yeal Ha, and Insoon Yang
Abstract: In this paper, we propose a consensus-based algorithm for nonconvex optimization on the Stiefel manifold. For a given objective function on the Stiefel manifold, we construct a stochastic interacting particle system for sample points so that all the sample points are expected to asymptotically converge to a single point, which is close enough to a global minimizer. We show the global existence and uniqueness of solutions to our stochastic differential equation (SDE) model for consensus. A predictor-corrector type numerical scheme is then proposed for implementing the SDE model with the guarantee that each sample point stays on the Stiefel manifold. A salient feature of our algorithm is that it is gradient-free, thereby applicable to a wide range of problems. The results of our numerical experiments demonstrate that the proposed method can successfully find a global minimizer even when the objective function is nonconvex.