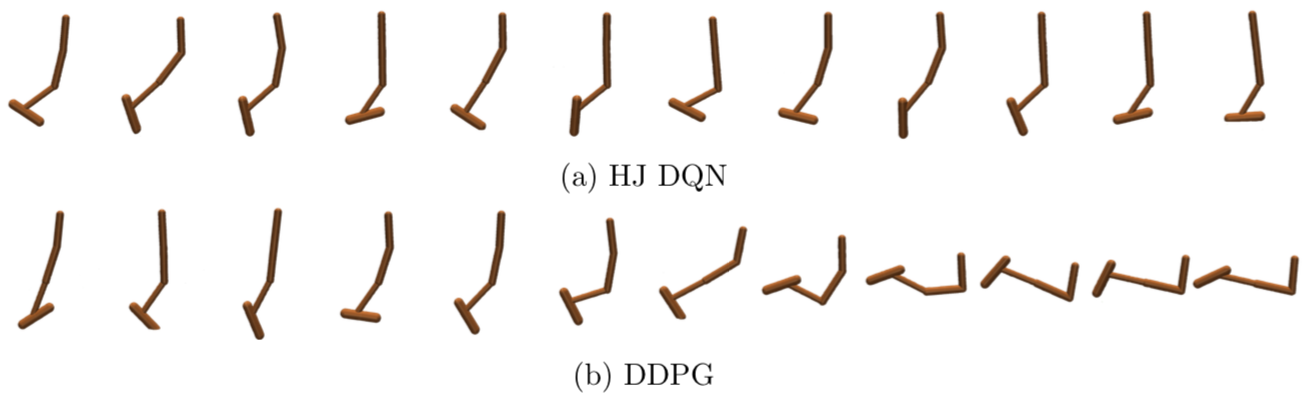
The paper “Hamilton-Jacobi deep Q-learning for deterministic continuous-time systems with Lipschitz continuous controls” has been accepted for publication in the Journal of Machine Learning Research (JMLR). It aims to extend the idea of deep Q-networks (DQN) to the continuous-time deterministic optimal control setting with Lipschitz continuous controls. A new class of Hamilton–Jacobi–Bellman (HJB) equations is derived to design our deep Q-learning algorithm without requiring actor networks or numerical solutions to optimization problems for greedy actions; the HJB equation provides a simple characterization of optimal controls via ordinary differential equations.
Hamilton-Jacobi deep Q-learning for deterministic continuous-time systems with Lipschitz continuous controls
by Jeongho Kim, Jaeuk Shin, and Insoon Yang
Abstract: In this paper, we propose Q-learning algorithms for continuous-time deterministic optimal control problems with Lipschitz continuous controls. A new class of Hamilton–Jacobi–Bellman (HJB) equations is derived from applying the dynamic programming principle to continuous-time Q-functions. Our method is based on a novel semi-discrete version of the HJB equation, which is proposed to design a Q-learning algorithm that uses data collected in discrete time without discretizing or approximating the system dynamics. We identify the condition under which the Q-function estimated by this algorithm converges to the optimal Q-function. For practical implementation, we propose the Hamilton–Jacobi DQN, which extends the idea of deep Q-networks (DQN) to our continuous control setting. This approach does not require actor networks or numerical solutions to optimization problems for greedy actions since the HJB equation provides a simple characterization of optimal controls via ordinary differential equations. We empirically demonstrate the performance of our method through benchmark tasks and high-dimensional linear-quadratic problems.